Q&A
How Improbable helps militaries by modelling defence operations
Working with various research institutions and universities, Improbable Defence explores the potential of simulation modelling in defence applications. Norbert Neumann finds out more from the company's chief executive officer of defence and security, Joe Robinson.
Simulations to predict outcomes of scenarios have been used in a number of areas, most prominently during pandemics and endemics to inform policies and help decision-makers put certain protective measures.
UK digital infrastructure specialist Improbable Defence and its research team believe that methods used in such simulations also could - and should - be used in defence. Improbable Defence chief executive officer of defence and security Joe Robinson tells us more.
Norbert Neumann:
What methods could you use for modelling in a defence context, and what role does technology play in this?
Joe Robinson:
Modelling and simulation is becoming more pervasive throughout the defence environment as applications are expanding, and their associated techniques can be applied across the full spectrum of functional activities of armed forces.
Methodologies like time-series models, widely used for commercial or environmental forecasting, are repurposed, for example, to investigate the behaviour of military flight parameters and pilot performance over time and help direct future mission planning faster.
With vast amounts of data becoming ever-more available, however, other methods such as agent-based models also come to play, helping create simulations of actions and interactions of autonomous agents that produce behavioural patterns.
The application of modelling and simulation across all defence operational domains has become an important aspect of delivering efficient and effective military operations in areas such as military training, operational analysis, rapid prototyping, doctrine development, mission planning.
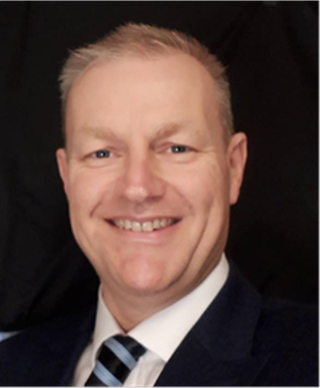
// DSEI space advisor Dr Michael Holden
Could you explain what such a simulation would look like?
Simulations based on computational models combine artificial intelligence (AI) and machine learning with trusted data sets from multiple sources to support and create detailed, credible and realistic representations of real-life scenarios troops may face in the modern battlefield.
In the case of Improbable Defence’s synthetic environment, data sets can take the form of a 3D digitally rendered city, region or country with high-quality mapping and information about critical infrastructure such as power, water, transport and telecommunications.
The multi-layered environment can also include a populace of AI agents who simulate behaviours and political sentiment based on natural language processing (NLP) technology, a type of machine learning that reads vast amounts of text data such as news and intelligence reports. This can then be overlaid with other information, such as smart-city data, information about military deployments or data gleaned from social listening.
By synthesising the complexities of the modern, multi-domain operating environment, users can interact directly with the virtual environment in an immersive training experience which keeps decision-makers informed in real time, helps them identify concerns or threats early and enables them to explore their response options along with the potential consequences of each different action.
There are estimates that we've got something like four to five times the level of private investment in space capabilities than we do in government, which is a huge turnaround from a decade ago.
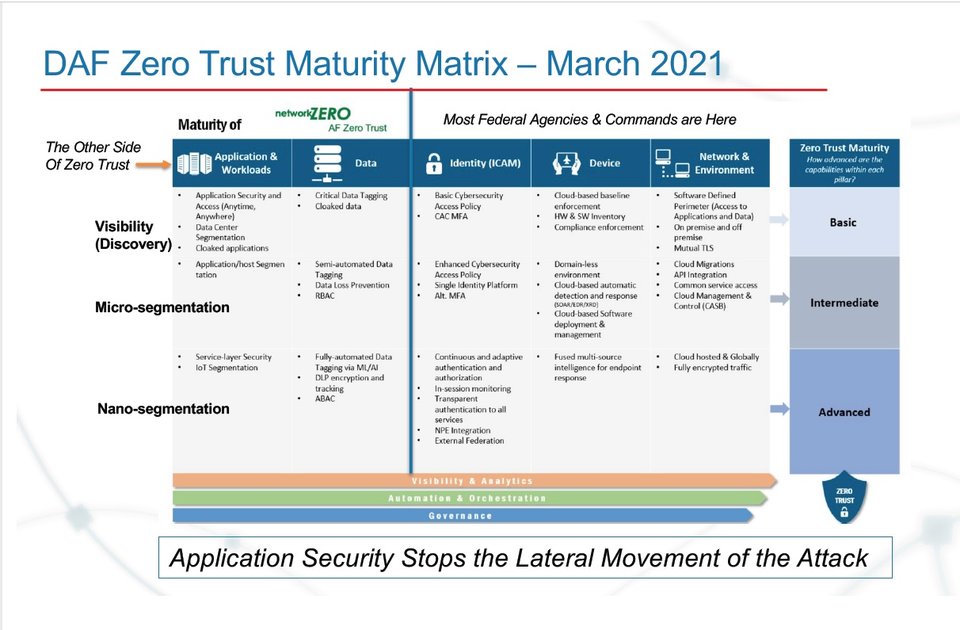
// MORFIUS is a high-powered microwave-based interceptor. Credit: Lockheed Martin
How can you ensure reliability and high fidelity in these simulations?
Data exploitation is dependent on the quality of information which is essential to create models that can reliably reflect the real world and give a rich, detailed picture of how, for example, a region or city might react to a given event: a military operation, an epidemic or a cyberattack, or a combination of such events.
Ample information can be collected from intelligence reports, open sources like social media and news reports and trusted partners across academia which NLP technologies can digest to draw an accurate depiction of reality. Credible data is also owned by critical institutions and government organisation who can add an extra layer of reliability. However, machine learning techniques need to be tuned and adjusted to the available data and the problem in question to ensure peak performance.
Can you elaborate on the concept of “active learning”?
Active learning, in the context of this work, is a kind of experimental design strategy. It can be contrasted with, for example, standard Monte Carlo methods wherein many parameter values are drawn from a fixed distribution, after which the model is run on all of them and the resulting model outputs scrutinised.
In active learning, on the other hand, one would sample a single parameter value, run the associated model, and allow the choice of the next parameter value to depend on the previously seen model outputs: with each new observation we learn something new and change our approach as a result.
This strategy is particularly appealing when there is likely to be an area of the parameter space that requires more computational effort (for example, the region in which some loss function of interest is minimised) but it isn’t known in advance of doing experiments where that area will be. It could enable machine learning to help our customers make operational decisions.
How is active learning able to produce more realistic simulations than others?
We’d like to push back on the use of ‘realistic’ here as it is often misinterpreted - we’re not trying to emulate the world. Simulation is about usefulness to the task at hand - whether that be trainees achieving training outcomes in virtual exercises, or decision-makers making more robust and explainable choices. Our approach seeks to address three core problems:
Firstly, greater interconnection in the world has led to ever deeper interdependence between economies, institutions and all manner of critical socio-technical systems across regions and nations.
Traditional disciplinary thinking is often too limited to support adequate understanding of this densely woven fabric and to support effective decision-making. Our technology enables ecosystems of interconnected data, artificial intelligence technologies, machine learning systems and constructive models such as digital twins and simulators.
Our synthetic environments have the breadth to understand inter-system consequences, the depth and detail to explore the consequences, and can scale to cover extensive areas of interest.
Secondly, in order to react quickly and effectively, joined up processes are required that span tools and job functions. The insight produced by an analyst using a machine learning application, for example, needs to be compiled with other sources, reviewed, planned against and acted upon.
This requires a connected and interdependent suite of technological capabilities such as cloud computing, data storage, modelling and simulation. Improbable’s platform brings these technologies together to realise the full potential value of synthetic environments.
Finally, the barrier to entry to fully utilise the apparatus of data-driven decision-making is often an advanced degree in a highly technical field. As quickly as tools become easier to use, data volumes grow, analytical methods evolve and complexity increases. To the greatest extent possible tools need to be accessible, straight forward to use and facilitate easy collaboration.
// Main image: Improbable Defence modelling simulation at DSEI 2021. Credit: Improbable Defence