The matrix below details the areas in AI where defence companies should focus their time and resources. They are advised to adapt existing AI commercial off-the-shelf (COTS) technology to military uses, remaining largely hardware agnostic and focusing on software.
Militaries, the end-users, will only invest in platforms and apps to adapt them to specialist needs. We suggest that defence companies invest in technologies shaded in green, explore the prospect of investing in technologies shaded in yellow, and ignore areas shaded in red.
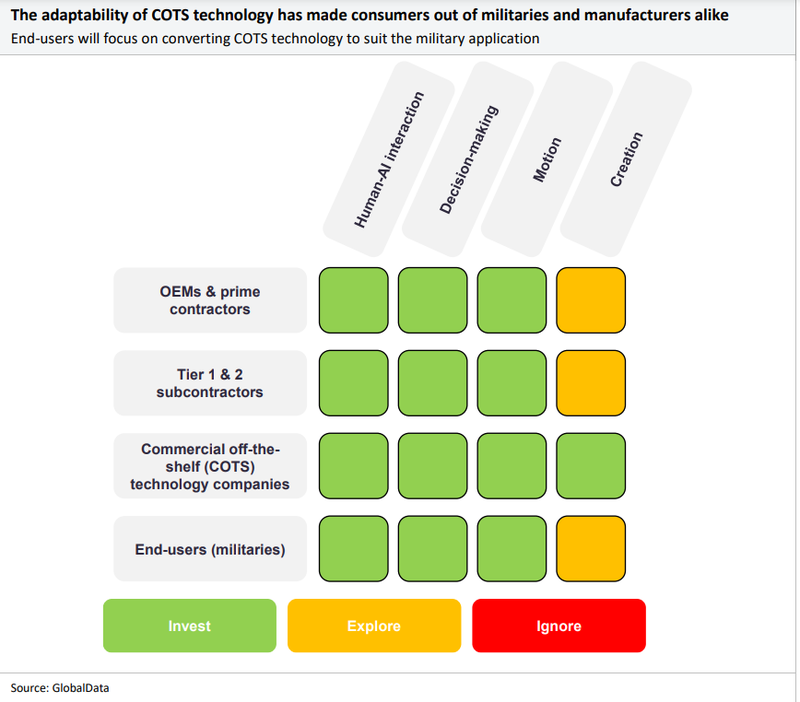
Rising geopolitical tensions demand that military organisations and the defence industry modernise all aspects of equipment and operations to meet the evolving needs of modern armed conflict. As such, no major player within the defence supply chain can afford to ignore any AI value chain layer; however, they should prioritise specific value chain layers.
Original equipment manufacturers (OEMs) and prime contractors must invest in human-AI interaction, decision-making, and motion and explore the creation layer. Investment in the human-AI interaction layer is crucial as it encompasses conversational AI, brain-machine interfaces, other sensory AI, and computer vision.
The defence sector has some of the most stringent manufacturing specifications of any industry. Computer vision will automate and enhance quality control throughout the manufacturing process. Investment in decision-making will also enhance manufacturing by accurately planning and forecasting supply, demand, and manufacturing time; motion will aid in automating manufacturing and logistics.
Finally, OEMs should explore the creation layer and the use of generative AI in the design phase of products and components.
Tier 1 and 2 subcontractors must also invest in the human-AI interaction, decision-making, and motion layers and explore the creation layer. Tier 1 and 2 subcontractors would have similar reasonings as OEMs and prime contractors as both are defence manufacturers, albeit at different scales.
COTS companies are involved across all layers of the AI value chain and should, therefore, invest in all layers. COTS companies have become the major suppliers to defence manufacturers and militaries alike.
Militaries are the ultimate end user and should invest in the human-AI interaction, decision-making, and motion layers and explore the creation layer. Computer vision from the human-AI interaction layer is well suited to automating C4ISR analysis.
Investment in the decision-making layer will further enhance automated C4ISR analysis, threat analysis, and decision-to-fire capabilities in lethal autonomous weapons.
Investment in the motion layer is critical for the full realisation of lethal autonomous weapons which can operate in the air and on land, and in maritime and submarine operations. Finally, militaries should explore using generative AI (creation layer) for potential use in procedurally generated content for training and simulation purposes.
However, as with current simulators, this will primarily be provided by third-party specialist companies, not developed in-house by the military.
How AI addresses the challenge of AI ethics
The lack of explainability of many AI algorithms raises major ethical concerns, especially if these algorithms control multimillion-dollar pieces of lethal military hardware. Understanding the decision-making process underlies our ability to trust AI, but a lack of transparency undermines confidence in this technology.
Explainable AI models will go some way to restoring that confidence; it refers to an AI system that allows humans to understand how the AI arrives at a decision and offers explanations for its decision-making process.
How AI addresses the challenge of cybersecurity
AI is often considered a double-edged sword in the field of cybersecurity. As AI capabilities continue to evolve, it is becoming a tool for both protecting against cyberattacks and launching them. New autonomous technologies can increase cyberattacks' anonymity and allow them to be executed at a much larger scale and faster speeds. AI can accelerate malware, allowing code to change constantly, thus making it more difficult to detect. Future AI techniques could allow hackers to bypass facial security and spam filters, promote fake voice commands, and avoid anomaly detection engines.
Securing systems against such attacks is critical as breaches can offer attackers access to vast amounts of data which could pose an immediate risk to military operations. An AI-enabled defensive approach allows cyber teams to stay ahead of the threat as machine learning (ML) technology improves the speed and efficacy of both threat detection and response, providing greater protection.
ML solutions can boost threat detection accuracy and authorise instant autonomous responses to react to new AI-generated cyberattacks. This wave of innovation is considered a more proactive approach to security.
How AI addresses the challenge of the data deluge
The realm of C4ISR is a natural home for AI, requiring both precision and endurance. Potential applications include UAVs or loitering munitions flying over hostile areas to send relevant intelligence to their command centre, facial recognition for surveillance, autonomous ground vehicles patrolling porous borders around the clock, and UAVs surveillance of remote areas of operations.
Using AI for C4ISR missions protects humans from the dangers of a hostile environment without sacrificing efficiency. If anything, AI will eventually be able to perform these types of missions more effectively than human operators.
AI-assisted intelligence analysis can aid in deriving insights from large datasets. For example, the US Department of Defense’s leading AI initiative, Project Maven, aims to use computer vision algorithms to sift through the vast amounts of footage collected by UAVs and automatically identify hostile activity. Within the field of ISR, AI is intended to automate the work of humans, who currently spend hours scanning through drone footage for actionable information.
For frontline roles, the plethora of sensors embedded in AI-enabled devices would contribute to the data deluge; however, these devices display information in an easily digestible format. This allows users to access essential data when needed, ensuring they can make faster and more informed decisions.
The role of AI in enhancing multi-domain operations is being explored as part of the US military’s Joint All Domain Command & Control (JADC2) initiative. The US Army successfully tested the integration of AI and commercial remote sensing satellite data into the ‘kill chain’ during Project Convergence 2020, highlighting the immediate impact these capabilities can have on the modern battlefield.
How AI addresses the challenge of maintenance, repair, and overhaul (MRO)
Logistics is a domain where defence manufacturers and armed forces are equally eager to see AI deliver optimisation. The potential cost reductions and efficiency improvements are attractive, both from a commercial and a military point of view.
Some defence contractors are already working on embedded AI components capable of assessing a platform's maintenance condition. Predictive logistics could also determine future spare parts and supply requirements, making the supply chain more reactive and cutting costs with just-in-time production.
Predictive maintenance is an AI-enabled technique that uses data analysis tools and techniques to detect anomalies in equipment and processes so that they can be fixed before they fail. A big chunk of most defence budgets is reserved for maintaining and servicing existing defence equipment.
By using predictive maintenance, the associated service costs of machinery can be reduced significantly. Estimates suggest that roughly 10% to 20% of operational costs can be reduced by implementing predictive maintenance.
AI-powered applications can predict subsystem failures, provide root cause analysis, and forecast part demand to ensure that maintenance is carried out at the right time and place, with the right parts at hand. Militaries would benefit from reduced unscheduled maintenance, optimised maintenance schedules, reduced down-time awaiting parts, and reduced in-flight aborts and emergency landings.
A prime example is GE Aviation’s GE90 engine, of which the firm created an AI-enabled digital twin to improve the maintenance of an aircraft engine blade. Over time, blades can experience what the aviation industry calls spallation, in which materials begin to erode.
GE customers believe that, using digital twins, they can better predict how a blade will degrade over time, allowing them to perform maintenance before a problem occurs.
How AI addresses the challenge of manned-unmanned teaming
AI is increasingly viewed as the most important emerging technological trend within the defence sector, as it would have multiple beneficial applications at the operational and tactical levels, particularly within the context of soldier modernisation.
One of the major advantages of increased automation is that it facilitates the implementation of various manned-unmanned teaming (MUM-T) concepts currently being developed to suit the operational requirements of various militaries and service branches. MUM-T entails the synchronised employment of human operators or manned platforms and unmanned vehicles, robotics, or sensors to achieve enhanced situational awareness, lethality, and survivability.
MUM-T plays an increasingly significant, mission-critical role in military aviation. It acts as a force-multiplier and provides the necessary capabilities to support often dangerous tasks over expansive areas of operations. Using the strengths of both manned and unmanned systems improves situational awareness, allowing for greater mission effectiveness and efficiency while affording greater safety and lower risk to human assets.
Providing pilots of manned aircraft with the ability to control unmanned air systems (UASs) enables them to take full advantage of the platform’s ISR capabilities to provide alternative data on potential threats, thus enhancing decision-making and improving safety during dangerous missions.
For soldiers, AI can rapidly analyse battlefield data, with the resulting information being processed and delivered using AR technologies to provide visualisation aids and assist with weapon targeting. The technology can be used to identify and classify threats, prioritise targets, and calculate distances. Such systems take information from multiple sensors across the battlefield to generate a complete picture.
Human soldiers will still control most military actions in the near term, but AI will provide easy-to-understand analysis and recommendations based on datasets that are too large for unaided humans to comprehend.
How AI addresses the challenge of training and education
Generative AI’s capacity to create and refine concepts using prompt-based coding can dramatically facilitate the development of digital simulation models and environments for virtual testing and training purposes. The further development of this technology could render virtual training more practical due to the heightened variation and accuracy in prompt-based simulated environments.
NASA has explored generative AI-based simulation models for experimental aircraft to identify the procedural benefits such systems could provide in developing future platforms or components.
GlobalData, the leading provider of industry intelligence, provided the underlying data, research, and analysis used to produce this article.
GlobalData’s Thematic Intelligence uses proprietary data, research, and analysis to provide a forward-looking perspective on the key themes that will shape the future of the world’s largest industries and the organisations within them.